First published on Erik Kolstads blog June 8. 2017
If you want to understand how seasonal forecasts are made, think of how you yourself make forecasts without even thinking about it. When you see a black cloud coming your way fast, you will start looking for shelter. The reason is of course that your brain tells you that there’s an outsized chance of rain.
A weather forecast model works much the same way. It’s a computer program that, if it knows the current state of the weather, it can predict what will happen next. That’s because it knows the laws of physics and chemistry that drive the winds, the rains and all the other components of the weather. If the information that is fed into the weather model – what we call the initial condition – is good, the prediction will be very accurate for the next few hours and days. A weather forecast many days ahead, on the other hand, is often inaccurate. There are mainly two reasons for this:
- The initial conditions fed into the model are incomplete. In practice, this information consists of all available observations, from weather stations around the globe, ships, weather balloons, ocean buoys, and not least: data from satellites up in space. They routinely scan the surface and the atmosphere using everything from microwaves and radar to regular images. In regions where the observational network on the ground is dense, such as in Central Europe and in large parts of North America and East Asia, forecasts are usually reliable. In the Arctic and in most of Africa, there are fewer observations, and the weather forecasts are not so good. The errors in the model due to poor initial conditions will grow with time, so that a forecast several days into the future gradually deviates from what actually happens.
- The motions in the atmosphere are somewhat chaotic. This means that there is a certain degree of randomness involved. Imagine that you want to predict how a drunk man moves in a desert or another wide open space. You know where he is when he starts walking, so you can predict the general area where he’ll be in 10 seconds. But (depending in how drunk he is) it’s harder to predict his whereabouts 10 minutes into the future. The atmosphere is not quite as random as that, but you get the idea. (One interesting point is that some people think that the atmosphere isn’t chaotic at all, it’s just that our models and the initial conditions will never be good enough. As a consequence, the atmosphere appears to be chaotic. The belief that something can be predicted given adequate initial conditions is called determinism and applies to the direction of our lives as well as physical phenomena.)
So, if weather forecasts several days ahead are unreliable, why should we trust seasonal forecasts, which predict the weather weeks and months into the future? We have to think about it differently. A weather forecast is usually absolute. It says: “It will rain tomorrow between 9 and 10 AM”. A seasonal forecast is more about probabilities. A typical forecast issued in May says: “There’s a high probability that June will be warmer than normal.”
The reason that seasonal forecasts are about probabilities is that they are based on several model simulations, not just one, as a regular deterministic weather forecast is. We accept that one particular forecast many days ahead will be imperfect. Therefore we perform many forecasts, feeding them slightly different initial conditions. All the forecasts are equally likely (or unlikely) to be correct, but at least we span a wider range of outcomes than with just one simulation.
Let’s consider an example. Say we have 50 weather simulations initialized based on observations in May. If 40 of these simulations indicate that June will be warmer than normal in a specific region, we could issue the forecast mentioned before (high probability of June being warmer than normal). If only 10 of the 50 simulations were warm, we would have to say that there was a low probability (only 20 %, in fact) of a warm June.
As it happens, the farther into the simulations one gets, the less they tend to agree. For a forecast issued in June, there will be few clear signals for August. The model simulations will be all over the place, unless there is an initial condition that is so momentous that it has an impact on all the model simulations far into the future. The El Niño phenomenon is one such feature.
In short, an El Niño episode is when the sea surface temperatures in parts of the tropical Pacific Ocean are higher than normal. This leads to massive heat loss from the ocean to the atmosphere, and it influences weather patterns far afield. For instance, the summertime rainy seasons in Ethiopia in East Africa is for a large part governed by El Niño, several thousand kilometres away.
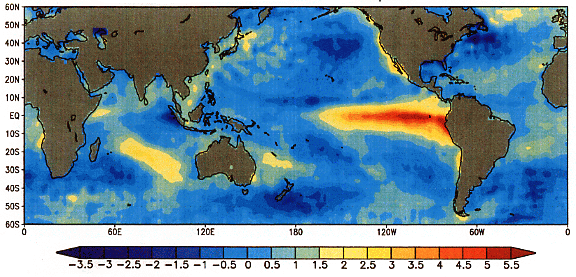
The El Niño phenomenon is a good example of the ocean acting as a good predictor of the atmosphere. The state of the ocean varies much more slowly than the winds and rains in the atmosphere. Therefore it is extremely important to represent the ocean (and the sea ice and snow cover in winter) as well as possible in order to produce a good seasonal forecast. The initial state of the atmosphere is not so important, as that will change drastically in just a few days anyway.
Seasonal forecasts can be very useful tools for planning. However, their quality is dependent on the quality of the initial conditions (observational data fed into the model), the number of simulations (it is expensive to perform many), and the state of the atmosphere and the ocean. In addition, the accuracy of the forecast is influenced by the current predictability of the ocean and weather system. For instance, if there is an El Niño going on, some regions are more predictable than normal.
What we climate scientists try to do, is to improve the seasonal forecasts by improving the models, enhancing the observational network, and not least: trying to understand why the atmosphere is sometimes more predictable than at other times. That’s our idea of fun.